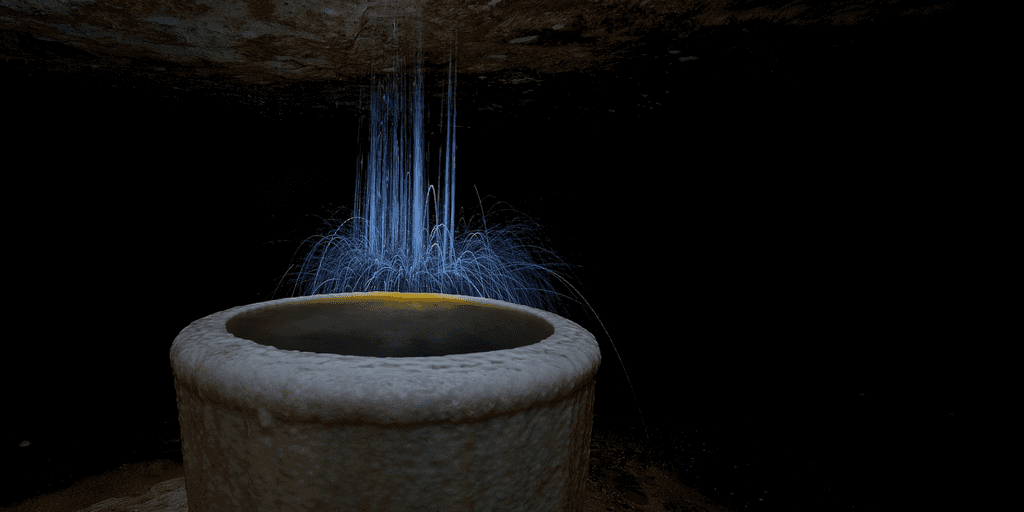
Hive, Calcite and Druid
By David WORMS
Jul 14, 2016
Do you like our work......we hire!
Never miss our publications about Open Source, big data and distributed systems, low frequency of one email every two months.
BI/OLAP requires interactive visualization of complex data streams:
- Real time bidding events
- User activity streams
- Voice call logs
- Network trafic flows
- Firewall events
- Application KPIs
Traditionnal solutions
- RDBMS (Mysql..): don’t scale, need caching but adhoc queries remain slow
- Key/value store (HBase…): quick but takes forever to compute (pre-materialization of data)
Context
- Created in 2011, open-sourced in 2012
- Built for interactive analytics
- +150 contributors as of 2016
Main Features
- Column oriented
- Batch & real-time ingestion
- Scalable
- Sub-second response time for time-based slice-and-dice
Persistent storage
- Segment files partitionned by date
- Segment should be around 1GB
- If larger, use smaller partitions
- Segment consist of: timestamp column, dimension (eg page, username, city), metrics (for aggregation) and indexes (to speed up the lookup)
Druid + Hive
- Indexing complex query result in Druid using Hive
- Introduce a SQL interface to Druid
- Execute complex operations on Druid data
- Efficient OLAP queries in Hive
- Demo: based on Hive 2.20, no release plans yet, rely on Druid 0.9.1.1 and Apache Calcite 1.10.0, (master and awaiting 0.9.2)
Druid data source in Hive
How to index data in Hive, 2 ways
- Register Druid data sources in Hive when data already in druid
STORED BY 'org.apache.hadoop.hive.druid.DruidSTorageHandler' TBLPROPERTIES ("druid.datasource" = "wikiticker")
- Create druid data sources
STORED BY 'org.apache.hadoop.hive.druid.DruidSTorageHandler' TBLPROPERTIES ("druid.datasource" = "wikiticker" AS SELECT __time, page, user, c_added, c_removed FROM src;
“_time” is timestamp, “page, user” are dimensions, “c*” are metrics
Need to partition the data by date granularity.
Query Druid data sources from Hive
- Automatic rewriting with Calcite
- Use a Druid Input Format to fetch records into the Hive pipeline
- Will bypass the broker
Roadmap
- Push more computation to Druid (push down optimization)
- Parallelize results retrieval (bypass broker)
- Denormalize start schema
- Huge perspectives for materialized views in Hive with automatic input query rewriting