Databricks partner in Paris, France
Deploy end-to-end data ingestion platforms and Machine Learning applications.
Adaltas works with its customers to create unique solutions with the Databricks platform that help them accelerate innovation and productivity.
Databricks, founded by the original creators of Spark, Delta Lake and MLFlow, offers an open and unified platform for data and AI.
Spark is the de facto standard for Big Data processing. Delta Lake raises to a new level data warehouses and data lakes and helps enterprises to increase productivity and the reliability of their data storages. MLFlow helps enterprises manage their Machine Learning lifecycle, enabling data scientists to efficiently go from raw data to Machine Learning models in one platform.
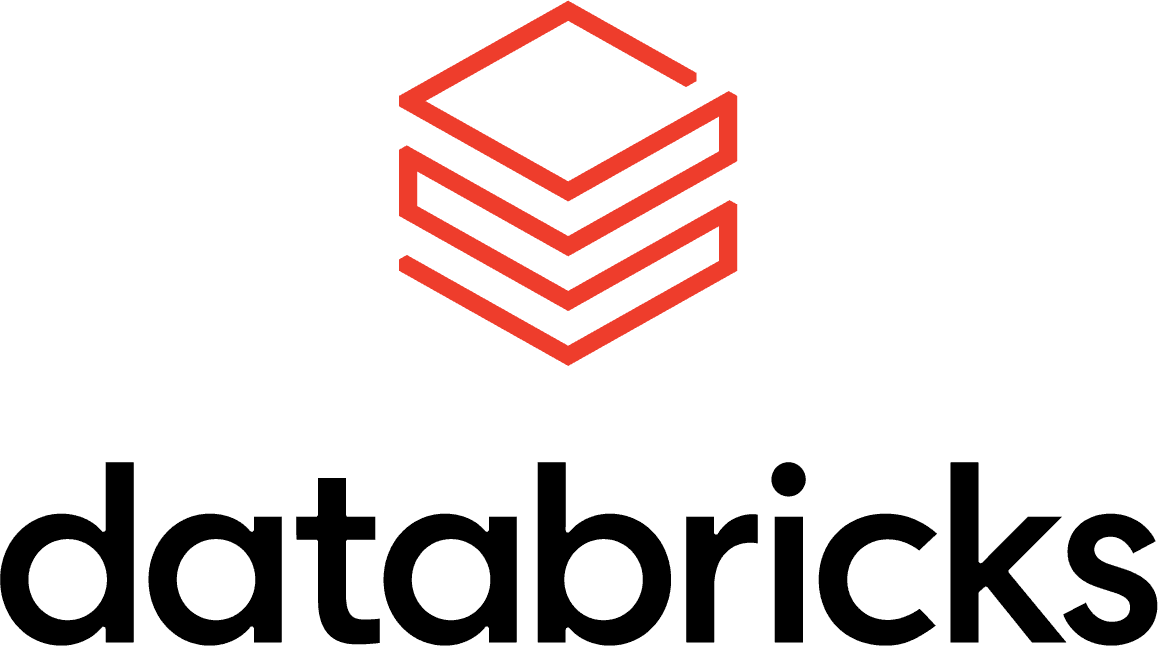
Discover Databricks with Adaltas
With the objective to promote Databricks in your company, we offer 2 days of consulting to our new customers.
Contact us for a detailed presentation of the Databricks platform and its potential impact in the context of your projects.
Build a practice
The Databricks platform removes the complexity of Big Data and Machine Learning. Your data teams composed of data engineers, data scientists and business leaders can now collaborate across all your workloads, accelerating your journey to become truly data-driven.
Transform your Big Data practice
- Build Databricks skills.
- Accelerate the time to value (TTV).
- Expand the value proposition for you Big Data & AI solutions.
Build a unified Analytics practice
- For data science, data engineering and analytical use cases.
- Accessible to technical and business users.
- Collaborate inside a compresensive platform.
Innovate with Big Data & AI
- Simplify the data architecture.
- Eliminate the data silos.
- Work across teams and innovate faster.
Methodology and roadmap for success
Adaltas works with your team to leverage the Databricks platform with a comprehensive Methodology. Our experts are certified with Databricks as well as with the major Cloud providers including Microsoft Azure, Amazon AWS and Google GCP.
Qualify the use case
- What is the business challenge today.
- What is the business outcome and value you are hoping to achieve.
Qualify the data
- Is the data in the cloud?
- Describe the data: type, size, format, speed, ...
- Understand the complexity of the Big Data the client is working with.
Qualify the solution
- Describe the current technology ecosystem and data pipeline architecture.
- Who are the data users? (data scientits, data engineers, business users)
State-of-the-art platform for analytics and AI in the cloud
The extensive Spark ML libraries and integration with popular frameworks such as Tensorflow, PyTorch, etc. make Databricks the market leader among AI platforms. Additionally, the introduction of MLFlow has made managing the machine learning lifecycle easy and productive.
Discover past work and don't recreate the wheel
- Building models is a very iterative process and most gains are incremental.
- Almost all Data Scientist teams regularly recreate work and therefore won't get as far as they could by refining past work. It is also a waste of money.
Collaboration between DS
- There is value to also sharing past work or working together on diffrent parts of the problem. Having a system of record for how work is done makes things easier and increase satisfaction.
- Collaborate with business users, data engineers and analyts.
Easy reproducibility of own and other works
- If a model is not reproducible, it is worthless.
- It is also a cornertone of collaboration. Two individuals need to be able to reproduce others results.
Articles related to Databricks
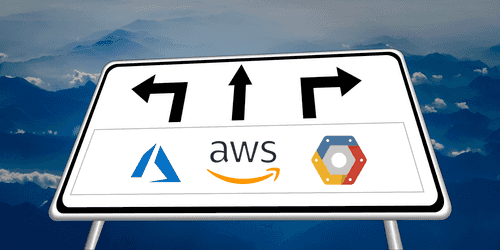
Should you move your Big Data and Data Lake to the Cloud
Categories: Big Data, Cloud Computing | Tags: DevOps, AWS, Azure, Cloud, CDP, Databricks, GCP
Should you follow the trend and migrate your data, workflows and infrastructure to GCP, AWS and Azure? During the Strata Data Conference in New-York, a general focus was put on moving customer’s Big…
Dec 9, 2019
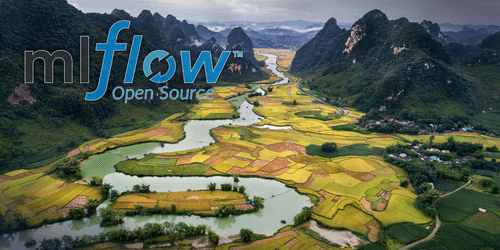
MLflow tutorial: an open source Machine Learning (ML) platform
Categories: Data Engineering, Data Science, Learning | Tags: AWS, Azure, Databricks, Deep Learning, Deployment, Machine Learning, MLflow, MLOps, Python, Scikit-learn
Introduction and principles of MLflow With increasingly cheaper computing power and storage and at the same time increasing data collection in all walks of life, many companies integrated Data Science…
Mar 23, 2020
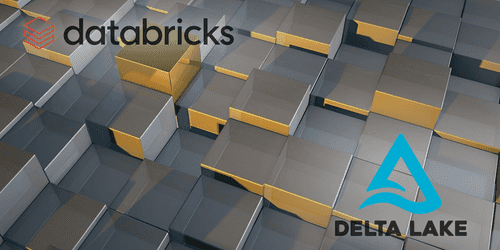
Importing data to Databricks: external tables and Delta Lake
Categories: Data Engineering, Data Science, Learning | Tags: Parquet, AWS, Amazon S3, Azure Data Lake Storage (ADLS), Databricks, Delta Lake, Python
During a Machine Learning project we need to keep track of the training data we are using. This is important for audit purposes and for assessing the performance of the models, developed at a later…
May 21, 2020
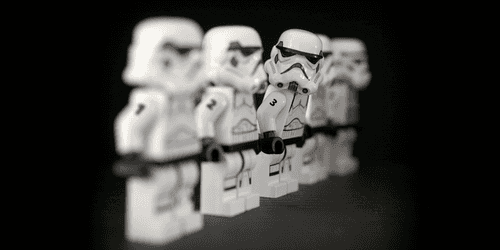
Version your datasets with Data Version Control (DVC) and Git
Categories: Data Science, DevOps & SRE | Tags: DevOps, Infrastructure, Operation, Git, GitOps, SCM
Using a Version Control System such as Git for source code is a good practice and an industry standard. Considering that projects focus more and more on data, shouldn’t we have a similar approach such…
By Grégor JOUET
Sep 3, 2020
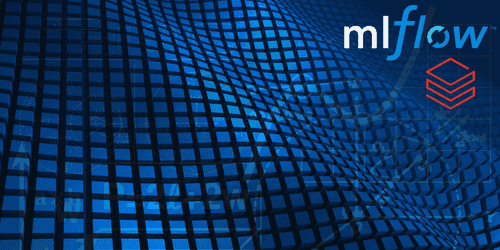
Experiment tracking with MLflow on Databricks Community Edition
Categories: Data Engineering, Data Science, Learning | Tags: Spark, Databricks, Deep Learning, Delta Lake, Machine Learning, MLflow, Notebook, Python, Scikit-learn
Introduction to Databricks Community Edition and MLflow Every day the number of tools helping Data Scientists to build models faster increases. Consequently, the need to manage the results and the…
Sep 10, 2020
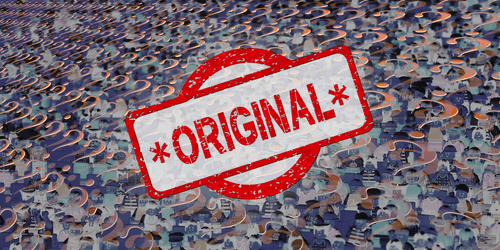
Data versioning and reproducible ML with DVC and MLflow
Categories: Data Science, DevOps & SRE, Events | Tags: Data Engineering, Databricks, Delta Lake, Git, Machine Learning, MLflow, Storage
Our talk on data versioning and reproducible Machine Learning proposed to the Data + AI Summit (formerly known as Spark+AI) is accepted. The summit will take place online the 17-19th November…
Sep 30, 2020
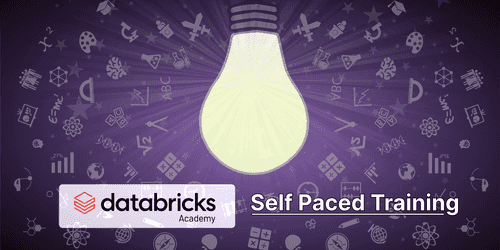
Self-Paced training from Databricks: a guide to self-enablement on Big Data & AI
Categories: Data Engineering, Learning | Tags: Cloud, Data Lake, Databricks, Delta Lake, MLflow
Self-paced trainings are proposed by Databricks inside their Academy program. The price is $ 2000 USD for unlimited access to the training courses for a period of 1 year, but also free for customers…
May 26, 2021
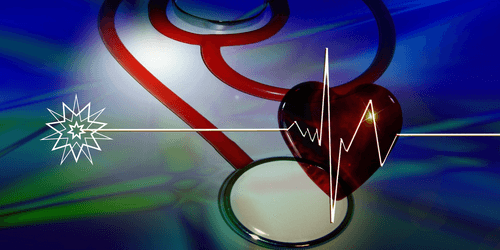
Databricks logs collection with Azure Monitor at a Workspace Scale
Categories: Cloud Computing, Data Engineering, Adaltas Summit 2021 | Tags: Metrics, Monitoring, Spark, Azure, Databricks, Log4j
Databricks is an optimized data analytics platform based on Apache Spark. Monitoring Databricks plateform is crucial to ensure data quality, job performance, and security issues by limiting access to…
By Claire PLAYE
May 10, 2022
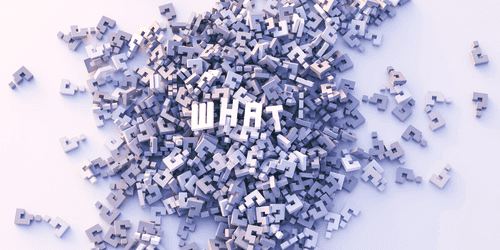
Data platform requirements and expectations
Categories: Big Data, Infrastructure | Tags: Data Engineering, Data Governance, Data Analytics, Data Hub, Data Lake, Data lakehouse, Data Science
A big data platform is a complex and sophisticated system that enables organizations to store, process, and analyze large volumes of data from a variety of sources. It is composed of several…
By David WORMS
Mar 23, 2023